Epi Info™ User Guide
StatCalc
How to: Analysis of Single and Stratified Tables
These tables are similar to those produced in Classic Analysis. Both single and stratified 2-by-2 tables can be analyzed to produce odds ratios and risk ratios (relative risks) with confidence limits, several types of chi square tests, Fisher exact tests, Mantel-Haenszel summary odds ratios and chi squares, and associated p values
Assumptions
- The values in the cells must be counts representing the number of records meeting the specifications in the marginal and stratum labels.
- A case-control study is one in which the ill and well individuals are selected and the number of exposed and unexposed is subsequently ascertained. In a cohort study, the exposed and unexposed are selected and the number of ill in each group is subsequently ascertained. A cross-sectional study starts with neither illness nor exposure determined, and ascertains both during the study.
- In cohort studies, the relative risk may be calculated from the results. In case-control studies, the odds ratio may be used as an approximation of the relative risk if the disease is rare in the general population from which cases and controls are selected. Fewer than one case in 20 individuals might be taken as a starting point. Thus, in a foodborne outbreak, selecting cases and controls from those who ate at a particular restaurant in a given week, the odds ratio could not be used to approximate relative risk if half of the individuals became ill. The odds ratio would, however, be an indicator of the degree of association between illness and the consumption of a particular food.
- For the results to be valid, the outcomes in each record must be independent of those in other records. The values for one individual do not predict those for another. Confounding must be removed by stratifying on confounding variables.
Single 2-by-2 Tables
Two-by-two tables are frequently used in epidemiology to explore associations between exposure to risk factors and disease or other outcomes. The table in StatCalc has Exposure on the left and Disease across the top. Not all textbooks and articles use the same conventions. Carefully observe the labels and transpose data items if necessary.
Given a yes-no or other two-choice question describing disease and another describing exposure to a risk factor, StatCalc produces several kinds of statistics that test for relationships between exposure and disease. Generally, an association is suggested by an odds ratio or relative risk larger or smaller than 1.0. The further the odds ratio or relative risk is from 1.0, the stronger the apparent association. Statistical significance can be assessed by p-values for the Chi square tests that are small <.05 if often used, Fisher exact test with a small p value; or confidence limits for the odds ratio that do not include 1.0.
The expected value of a cell is the product of the marginal totals for that cell divided by the grand total for the table. If any expected value is less than five, it is recommended you use the Fisher Exact test results and the Exact confidence limits. If the numbers in the table are all large, the other tests should indicate nearly the same result.
Stratified Analysis of 2-by-2 Tables
If confounding is present, associations between disease and exposure can be missed or falsely detected. A confounding factor is one that is associated with the disease and the exposure, but may not be of interest or observed. Age is a frequent confounder, although any factor other than the main exposure being considered can be treated as a confounder.
Stratification means making a separate table of disease by exposure for each possible confounder combinations. In the simplest case, this could mean separate male and female tables if sex is the potential confounder. If Age, Sex, and City are confounders, separate tables would be made for each possible combination of age group, sex, and city.
The Mantel-Haenszel weighted odds ratio, relative risk, summary Chi square, and p-value combine results from different strata to remove confounding caused by the variables used for stratification. If tables are entered for male and female, confounding by sex is removed. The degree of confounding can be judged by comparing the crude and weighted odds ratios; if they are identical, there was no confounding by sex.
The Approximate (Cornfield and Greenland/Robins) and Exact confidence limits provide additional measures. If the weighted odds ratio or relative risk (not for case-control studies) has confidence limits that do not include 1.0, there is a statistical association with 95% confidence between the disease and the exposure without confounding by the stratifying factor.
If the odds ratio or relative risks for strata in a series of stratified tables for the tests are not similar, then interaction between the stratifying factor and the risk factor are present. Logistic regression or other multivariate methods may be indicated (or the number of subjects is too small to draw definite conclusions). Alternatively, it may be advisable to present the stratum-specific estimates separately.
Experts in logistic regression recommend you thoroughly explore the data using stratified analysis before undertaking the regression analysis. If the number of confounding factors is fairly small and the odds ratios are homogeneous from stratum to stratum, stratified analysis may be all you need.
Example
A study of bladder cancers cases used randomly selected controls to explore the history of artificial sweetener use in the two groups.
Bladder Cancer |
||
Sweetener |
Yes |
No |
Ever Used |
1293 |
2455 |
Never Used |
1707 |
3321 |
- From the StatCalc application main page, select Tables <2 x 2, 2 x n>. A blank table opens in the StatCalc application window.
- Enter the data from the above sample table.
- Results will be generated as the different values in the cells are populated.
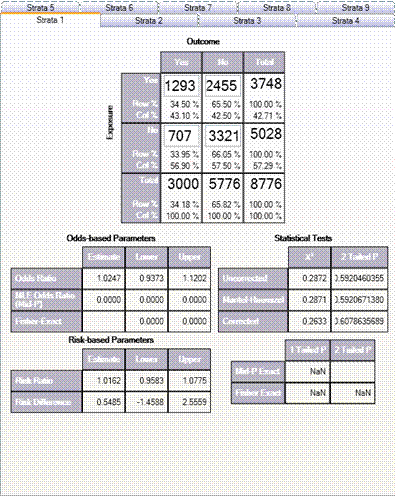
The odds ratio of 1.02 and the confidence limits that include 1.0 fail to provide evidence of any association between sweetener use and bladder cancer (cited by Schlesselman, p. 38-39).
Example
Relationship Between Alcohol Consumption and Myocardial Infarction (MI): Confounding Due to Smoking Hypothetical Data from Schlesselman, p. 182
The following case-control study indicates an apparent association between alcohol consumption and MI with an odds ratio of 2.26.
MI | |||
Alcohol |
Yes |
No |
|
Yes |
71 |
52 |
|
No |
29 |
48 |
Smoking is known to be associated with MI and alcohol consumption. Stratifying the data by smoking status creates two tables, one for smokers, and one for nonsmokers.
Nonsmokers | Smokers | ||||||
MI |
|
|
MI |
|
|||
Alcohol |
Yes |
No |
Alcohol |
Yes |
No |
||
Yes |
8 |
16 |
Yes |
63 |
36 |
||
No |
22 |
44 |
No |
7 |
4 |
The odds ratio for each table is 1.0, and the Mantel summary odds ratio is 1.0. The crude odds ratio and the Mantel summary odds ratio are quite different (4.0 and 1.0), concluding that smoking was a confounding factor and there appears (with this over simplified analysis) to be no association (odds ratio= 1.0) between alcohol and MI. Note that the odds ratio in the two strata are the same (1.0); there is no interaction or effect modification between smoking and alcohol. In other words, the effect of alcohol on MI is the same for smokers and nonsmokers. When the effect varies in the different strata (the odds ratios are different), interaction or effect modification is present.
To view and create a Stratified Analysis Summary of 2-by-2 Tables, take the following steps:
- From the StatCalc application main page, select Tables <2 x 2, 2 x n>. A blank table opens in the StatCalc application window.
- Enter the data from the above Nonsmokers sample table in the first Strata tab (Strata 1).
- Click on Strata 2
- Enter the data from the above Smokers sample table.
- The Stratified Analysis Summary of 2 Tables window gets populated as you enter data.
- Page last reviewed: December 23, 2015
- Page last updated: June 6, 2012
- Content source: